AI Integration: Key Advances in Smart Home Technology
Artificial Intelligence (AI) has the potential to transform the way we interact with our living spaces, making our homes smarter, more efficient, and incredibly responsive to our needs.
The integration of AI technologies such as machine learning, natural language processing, and computer vision is ushering in a new era of home automation that goes beyond simple programmable routines.
These AI-driven systems are capable of analysing vast amounts of data from various sensors and devices, learning from user behaviour, and making intelligent decisions to optimise comfort, energy efficiency, and security.
Let's examine innovative approaches where AI integration is enhancing smart home automation, focusing on the technical details that enable these improvements.
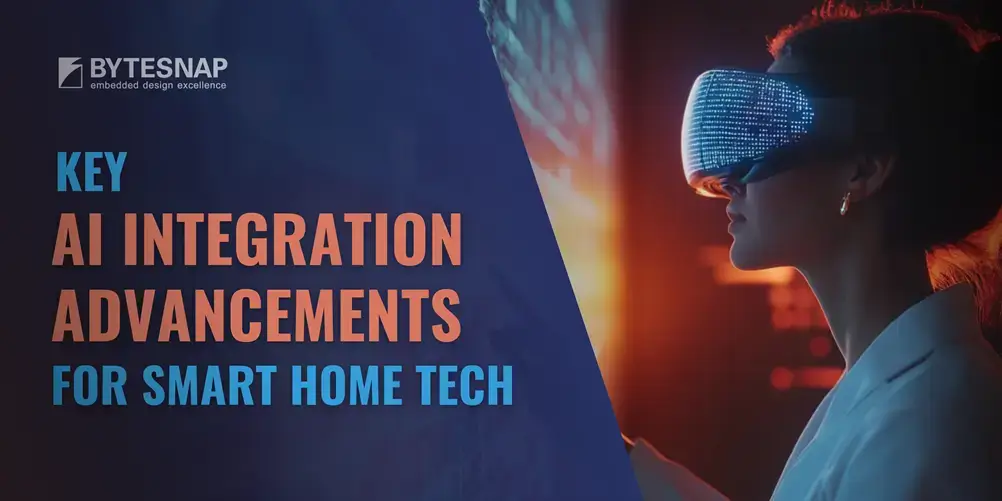
1. Enhanced Energy Management with AI-Powered Metering
AI-driven energy management systems are at the forefront of smart home innovation, offering unprecedented control over household energy consumption. These systems use advanced algorithms, including neural networks and reinforcement learning, to analyse usage patterns and optimise energy distribution throughout the home.
By leveraging techniques such as time series analysis and predictive modelling, these AI systems can forecast energy demand, adjust consumption in real-time, and even integrate with smart grids to participate in demand response programmes. The implementation of edge computing allows for rapid decision-making at the device level, reducing latency and improving overall system responsiveness.
These modules enable homeowners to monitor and manage their energy usage with precision, leading to significant cost savings and reduced environmental impact. The integration of machine learning algorithms allows for continuous improvement in energy prediction and optimisation strategies, adapting to changing household patterns and external factors such as weather conditions. Learn more about ByteSnap's energy solutions.
2. Intelligent Security Systems with Machine Learning
AI-based security systems are introducing new approaches to home monitoring. Machine learning algorithms are used to analyse data and potentially identify unusual patterns or events. The goal is to offer more sophisticated methods for detecting and responding to potential security concerns in residential settings. These systems utilise a combination of computer vision techniques, such as convolutional neural networks (CNNs) for image recognition, and anomaly detection algorithms to identify potential security breaches.
The implementation of federated learning techniques allows for continuous improvement of the security models while preserving user privacy. Additionally, the use of blockchain technology can enhance the integrity and immutability of security logs, providing a tamper-proof record of events. Discover more about ByteSnap's smart home security solutions.
3. Voice-Controlled Smart Home Assistants
AI-powered voice assistants have become the central hubs of many smart homes, offering intuitive control over various devices and systems. These assistants deploy sophisticated natural language processing (NLP) and natural language understanding (NLU) methods to interpret and carry out user instructions. The underlying AI models, often based on transformer architectures like BERT or GPT, are capable of understanding context, handling complex queries, and even engaging in multi-turn conversations.
These assistants leverage natural language processing and machine learning to:
- Understand and execute complex voice commands through sophisticated intent recognition and entity extraction algorithms
- Learn user preferences over time using reinforcement learning techniques, continuously adapting to individual speech patterns and command styles
- Integrate seamlessly with multiple smart home devices via standardised protocols and APIs, acting as a central control hub for the entire smart home ecosystem
- Provide personalised recommendations and automation through collaborative filtering and content-based recommendation systems
ByteSnap's expertise in developing voice control systems ensures that these AI assistants are both powerful and user-friendly. The implementation of on-device AI models, such as TensorFlow Lite or ONNX Runtime, allows for low-latency processing of voice commands while maintaining user privacy. The use of transfer learning techniques also enables rapid adaptation of voice models to different languages and accents, enhancing the global applicability of these systems. Explore ByteSnap's smart home solutions.
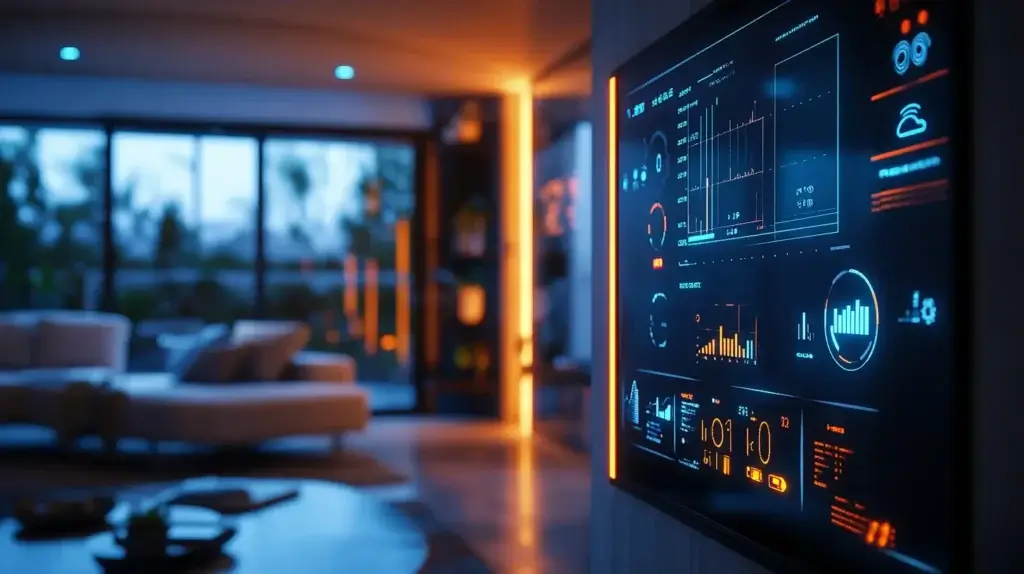
4. Personalised Automation with AI Learning
Machine learning algorithms enable home systems to tailor environments to each resident, interpreting patterns to anticipate needs and adjust settings accordingly.
These systems employ a variety of machine learning techniques, including reinforcement learning, clustering algorithms, and sequence prediction models, to understand and anticipate user behaviour.
By analysing patterns in device usage, environmental conditions, and user interactions, these AI systems can create highly tailored automation scenarios that evolve over time.ByteSnap's IoT and M2M device development services enable the creation of smart home systems that:
- Learn and anticipate user habits through advanced time series analysis and sequential pattern mining techniques
- Create customised automation scenarios using decision tree algorithms and fuzzy logic controllers for flexible rule creation
- Integrate multiple smart systems for seamless operation via standardised protocols like MQTT or CoAP, with semantic interoperability ensured through ontology-based approaches
- Adapt to changing user needs over time through online learning algorithms that continuously update the system's knowledge base
This level of personalisation ensures that each smart home is uniquely tailored to its occupants. The implementation of federated learning allows for collective improvement of personalisation algorithms across multiple households while preserving individual privacy.
Also, the use of explainable AI techniques provides transparency in decision-making, allowing users to understand and fine-tune the system's behaviour.
Discover ByteSnap's IoT and M2M solutions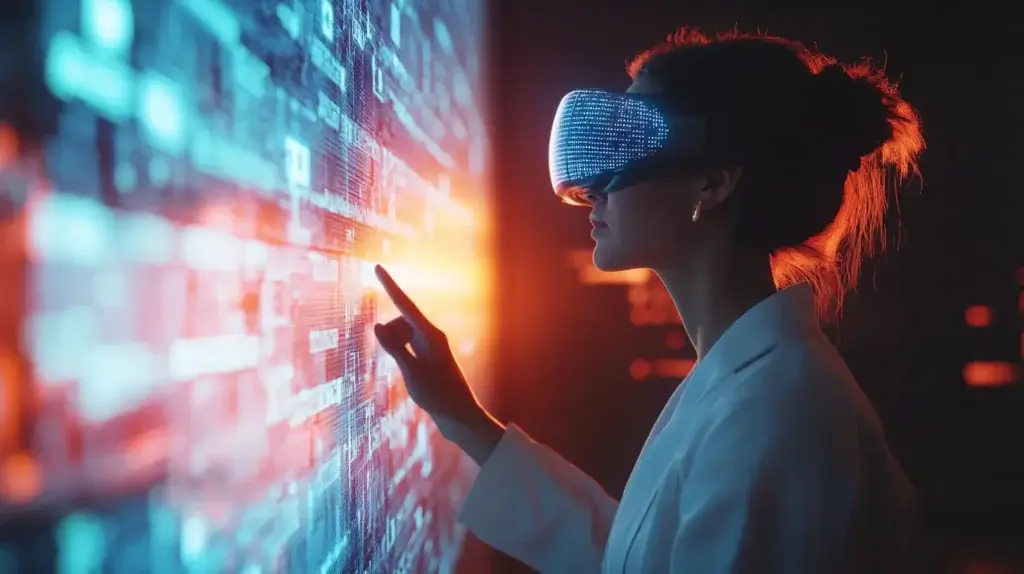
5. AI in Remote Health Monitoring
As populations age and chronic conditions become more prevalent, many individuals find themselves living alone while managing various health issues. This situation presents unique challenges:
- Increased risk of undetected health emergencies
- Difficulty in maintaining consistent health routines
- Potential for social isolation and its associated health risks
- Challenges in tracking and managing complex medication schedules
AI-powered remote health monitoring systems address these concerns by providing continuous, non-intrusive surveillance and support.
a) Continuous Data Collection and Analysis
AI systems can collect and analyse data from various sources:
- Wearable devices (smartwatches, fitness trackers)
- Smart home sensors (motion detectors, pressure pads)
- Voice-activated assistants
- Smartphone apps
These systems use machine learning algorithms to establish baseline health patterns for each individual and can detect anomalies that may indicate a health issue.
b) Predictive Analytics
Through the analysis of data patterns, AI can potentially identify early indicators of health concerns before they escalate into serious conditions. For example:
- Detecting changes in gait that might precede a fall
- Identifying irregular sleep patterns that could signal depression or other health problems
- Noticing changes in daily routines that might indicate cognitive decline
c) Emergency Response Activation
In case of detected emergencies, AI systems can automatically alert emergency services, family members, or caregivers. This rapid response can be life-saving in situations such as falls, heart attacks, or strokes.
d) Personalised Health Recommendations
AI systems can provide tailored health advice based on an individual's specific health profile and real-time data. This might include:
- Reminders to take medication or perform health-related tasks
- Suggestions for appropriate exercises or activities
- Dietary recommendations based on monitored health parameters
Benefits of AI-Powered Remote Health Monitoring
- Enhanced Independence: Individuals can maintain their autonomy while still receiving necessary support.
- Improved Quality of Care: Continuous monitoring allows for more accurate and timely interventions.
- Reduced Healthcare Costs: By preventing emergencies and promoting proactive health management, these systems can significantly reduce hospitalisation rates.
- Peace of Mind: Both the individuals and their families can feel more secure knowing that help is always at hand.
- Data-Driven Healthcare: The wealth of data collected can inform better treatment decisions and health strategies.
Conclusion: The Future of AI in Smart Homes
AI technology is gradually being incorporated into various aspects of smart home automation, from energy management to personalised experiences.Companies like ByteSnap Design are working on developing solutions to make our homes more intelligent and efficient. The combination of AI technologies such as machine learning and natural language processing with IoT devices is creating new possibilities for enhancing our living spaces.
The future of smart homes may involve further AI integration, with systems becoming more responsive to our needs. Some potential developments could include:
- Improved context-aware systems that can better understand and respond to occupants' preferences
- Health monitoring features that integrate data from various smart home devices to provide wellness insights
- Enhanced privacy and security measures to protect sensitive data while enabling AI capabilities
- Potential integration with smart city infrastructure, allowing homes to participate in broader urban management efforts
- More sophisticated decision-making abilities in smart home systems
As technology progresses, we may see smart homes become more capable assistants, helping to improve our quality of life in various ways. However, it's important to note that the full extent and timeline of these developments remain uncertain and will depend on ongoing research, technological advancements, and consumer adoption.
To stay ahead in the fast-moving smart home technology sector, explore ByteSnap's smart home solutions. Our team of engineering experts is continuously pushing the boundaries of what's possible in smart home technology, ensuring that our clients build the latest innovative solutions available into their product designs.
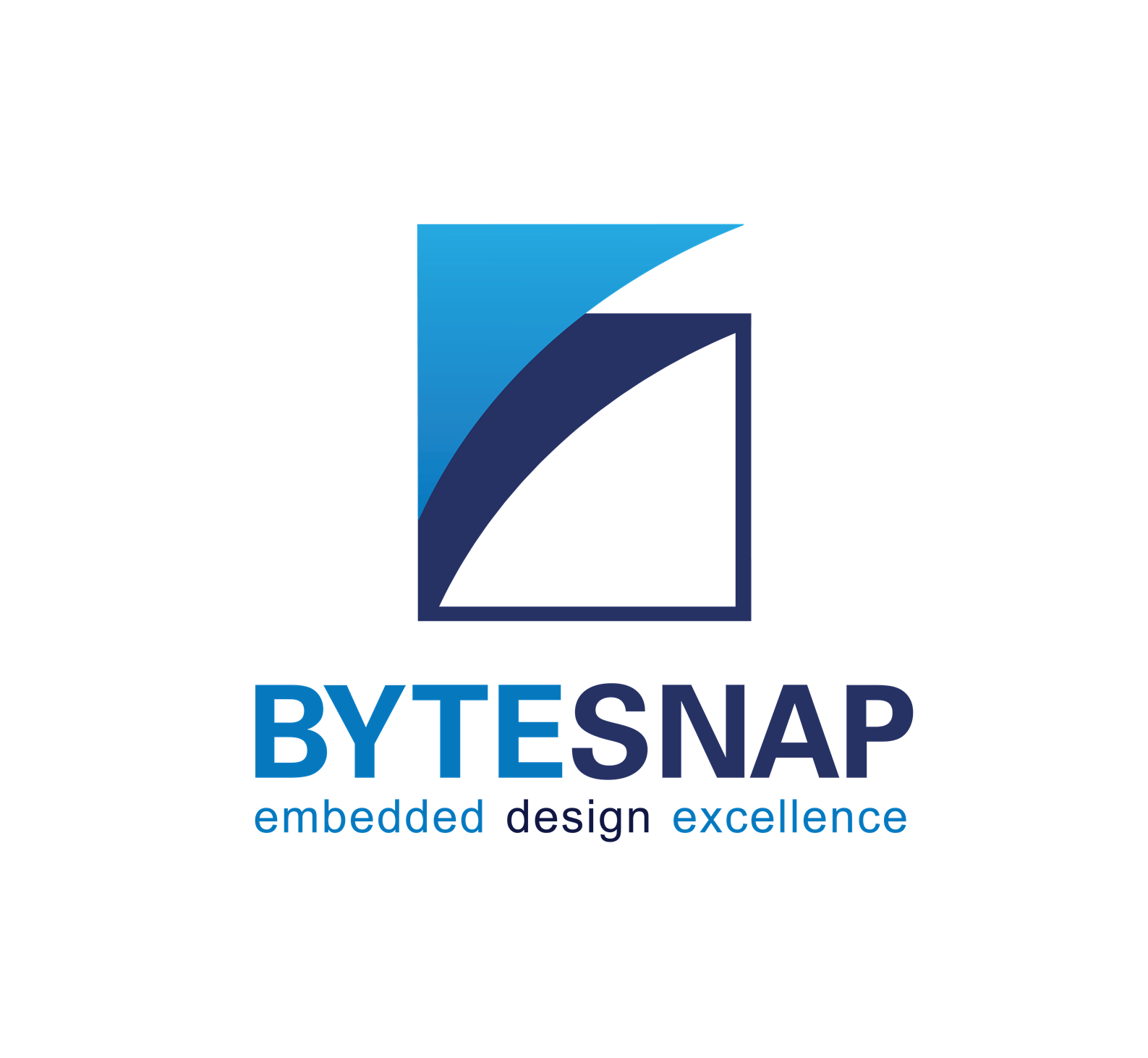
Founded in 2008, ByteSnap Design is an award-winning embedded systems design consultancy, offering a comprehensive range of services across the electronic product development lifecycle.
A highly skilled team of over 40 hardware and software engineers, our expertise spans several sectors, including IoT, automotive, industrial, medical, and consumer electronics.
The engineering consultants on the ByteSnap Editorial Team share their knowledge and practical tips to help you streamline your product development and accelerate designs to market successfully.
With their deep technical expertise and practical experience, they aim to provide valuable insights and actionable tips to guide you through the complex world of electronic product design and development, to help you bring innovative, reliable, and secure electronic products to market quickly and cost-effectively.